2013 Fantasy Baseball: OF Projections and Roto Ratings (#1 – #25)
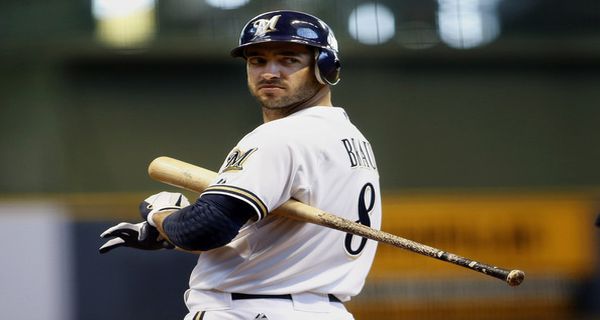
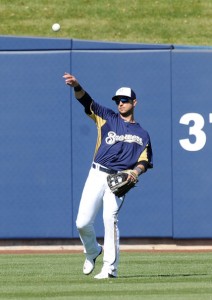
This is the fourth installment of our projection series. We are posting a series of articles in which we project the roto stats for all hitters who could be useful in mixed leagues. The projections and “Roto Ratings” for each player are available to those who subscribe to our premium content via our “Front Office” package. Today we have outfielders ranked #1 through #25. We will have #26 through #50 on Wednesday and #51 through #75 on Friday.
Big thanks to my buddy Brian Sager (@TheRealSAG) for helping me develop this idea and for talking through the whole thought process with me.
When analyzing a player’s Fangraphs page, you sort of automatically project the stats that you think the player will have in the upcoming season. But because you can’t memorize loose projections for 300+ players, you have to repeatedly go back to a player’s page and go through the mental process of projecting them again. But as a service to our premium content subscribers, I have decided to do the projections myself and make them available on the site.
The first step in the process is simply to project a range of possible outcomes for each player while assuming he plays a full season. To project those possible outcomes I use a variety of stats. To project batting average I factor in plate discipline skills (K%, BB%, Contact%, Swing%, Z-swing%, O-Swing%) and batted ball profiles (LD%, GB%, FB%). To predict home runs I again use the batted ball profiles as well as HR/FB rate from past years. To predict runs, RBI and steals I consider past performance in those categories, stolen base success rate, and where a player will be hitting in the lineup.
Every player can’t play every day, so you have to approximate how many games you think the player will miss and then fill in those games missed with the stats of the type of guy you might find on the waiver wire. To find that replacement level, I took the stats from the outfielders that were owned in less than 70% but more than 30% of ESPN leagues at the end of last year and averaged their stats. A replacement level guy in the outfield will give you the following stat line over the course of a season:
Category |
AVG |
HR |
SB |
R |
RBI |
Stats |
.270 |
14 |
12 |
59 |
54 |
The next step is to take the stats you think you’ll get from a player for the amount of games you project him to play once you factor in injury risk and playing time concerns. For example, I projected Josh Hamilton for 135 games, so I multiplied all my projections for him by 0.83 (135 games is 83% of 155 games). Then I took my replacement level stat line and multiplied all those numbers by the remaining 0.17. Then you add those two numbers together to get the final stat line you’d expect to get from 135 games from Hamilton and 20 games from a replacement level player. Hamilton’s projection after accounting for playing time and adding in a replacement player for his games missed looks like this:
Name |
G |
PA |
AVG |
HR |
SB |
R |
RBI |
Josh Hamilton |
155 |
676 |
.276-.284 |
30-34 |
5.0-8 |
92-96 |
98-104 |
135 |
589 |
0.279 |
30 |
7 |
89 |
95 |
After I got my final stat line, I decided to come up with a formula to use the projections to do rankings. This system I came up with is admittedly crude, but I think it does a pretty decent job of ranking the players.
I plan on ranking about 200 hitters, so I took the 200 hitters with the most plate appearances last season and created tiers for each roto category. For example, 20 guys hit above .307 last year. The next 20 guys hit between .293 and .306. So if I projected a guy to hit .308, I assigned him 10 points for average. If I projected him to hit between .293 and .306, I assigned him nine points, etc, etc. Because I projected ranges, I used the midpoint to see which tier someone fit into. I projected Ryan Braun to hit between .305 and .313. The midpoint there was .309. That fell within the first tier so I assigned Braun ten points for average. Below are the tiers I used:
AVG | HR | SB | R | RBI | |
10 | >.307 | >31 | >29 | >93 | >97 |
9 | .293-.306 | 25-30 | 20-28 | 86-92 | 86-96 |
8 | .286-.292 | 23-24 | 14-19 | 81-85 | 78-85 |
7 | .275-.285 | 19-22 | 11.0-13.0 | 74-80 | 72-77 |
6 | .270-.274 | 16-18 | 7.0-10.0 | 69-73 | 65-71 |
5 | .260-.269 | 14-15 | 5.0-6.0 | 65-68 | 59-64 |
4 | .250-.259 | 12.0-13.0 | 3.0-4.0 | 59-64 | 55-58 |
3 | .241-.249 | 9.0-11.0 | 2 | 54-58 | 49-54 |
2 | .229-.240 | 6.0-8.0 | 1 | 47-53 | 40-48 |
1 | <.229 | <6 | 0 | <46 | >39 |
After I assigned a player a point total for each individual category I added them all up and gave each player a score which I am calling their “Roto Rating.” Below are the projections and Roto Ratings for my outfielders ranked #1 through #25. Enjoy!
[am4show have=’p4;p7;p3;’ guest_error=’Front Office’ user_error=’Front Office’ ]
Name |
G |
PA |
AVG |
HR |
SB |
R |
RBI |
Total |
Ryan Braun |
155 |
681 |
.305-.313 |
34-38 |
25-29 |
102-107 |
110-114 |
|
10 |
10 |
9 |
10 |
10 |
49 |
|||
Mike Trout |
155 |
713 |
.298-.306 |
25-29 |
42-46 |
111-117 |
85-89 |
|
9 |
9 |
10 |
10 |
9 |
47 |
|||
Matt Kemp |
155 |
653 |
.293-.299 |
26-29 |
21-27 |
94-98 |
94-98 |
|
9 |
9 |
9 |
10 |
9 |
46 |
|||
Carlos Gonzalez |
155 |
663 |
.298-.305 |
28-31 |
19-23 |
91-95 |
94-99 |
|
142 |
608 |
0.299 |
28 |
20 |
90 |
93 |
||
9 |
9 |
9 |
9 |
9 |
45 |
|||
Andrew McCutchen |
155 |
665 |
.287-.293 |
25-28 |
18-22 |
90-94 |
94-98 |
|
8 |
9 |
9 |
9 |
9 |
44 |
|||
Justin Upton |
155 |
677 |
.284-.290 |
22-25 |
18-22 |
91-95 |
89-93 |
|
8 |
8 |
9 |
10 |
9 |
44 |
|||
Giancarlo Stanton |
155 |
642 |
.273-.279 |
41-44 |
5.0-6 |
85-89 |
102-108 |
|
148 |
613 |
0.276 |
41 |
6 |
86 |
102 |
||
7 |
10 |
5 |
9 |
10 |
41 |
|||
Jose Bautista |
155 |
672 |
.256-.264 |
43-46 |
8.0-10 |
96-101 |
98-103 |
|
148 |
642 |
0.261 |
43 |
9 |
97 |
98 |
||
5 |
10 |
6 |
10 |
10 |
41 |
|||
Adam Jones |
155 |
651 |
.281-.288 |
25-29 |
10.0-14 |
89-94 |
92-96 |
|
7 |
9 |
7 |
9 |
9 |
41 |
|||
Jason Heyward |
155 |
668 |
.266-.272 |
26-28 |
16-20 |
94-98 |
84-88 |
|
5 |
9 |
8 |
10 |
9 |
41 |
|||
Josh Hamilton |
155 |
676 |
.276-.284 |
30-34 |
5.0-8 |
92-96 |
98-104 |
|
135 |
589 |
0.279 |
30 |
7 |
89 |
95 |
||
7 |
9 |
6 |
9 |
9 |
40 |
|||
Matt Holliday |
155 |
669 |
.288-.294 |
24-27 |
3.0-4 |
90-94 |
97-101 |
|
8 |
9 |
4 |
9 |
10 |
40 |
|||
Yoenis Cespedes |
155 |
649 |
.283-.289 |
27-30 |
18-22 |
78-82 |
93-97 |
|
142 |
594 |
0.285 |
27 |
19 |
78 |
92 |
||
7 |
9 |
8 |
7 |
9 |
40 |
|||
Bryce Harper |
155 |
672 |
.274-.280 |
21-25 |
19-22 |
99-105 |
66-72 |
|
148 |
642 |
0.277 |
23 |
20 |
100 |
68 |
||
7 |
8 |
9 |
10 |
6 |
40 |
|||
Jay Bruce |
155 |
644 |
.252-.256 |
32-35 |
7.0-9 |
87-93 |
96-100 |
|
4 |
10 |
6 |
9 |
10 |
39 |
|||
Shin-Soo Choo |
155 |
703 |
.282-.290 |
16-20 |
19-23 |
97-103 |
70-74 |
|
148 |
674 |
0.285 |
18 |
21 |
98 |
71 |
||
7 |
6 |
9 |
10 |
6 |
38 |
|||
Austin Jackson |
155 |
698 |
.293-.297 |
14-17 |
13-17 |
108-112 |
63-67 |
|
148 |
667 |
0.294 |
15 |
15 |
107 |
64 |
||
9 |
5 |
8 |
10 |
5 |
37 |
|||
Alex Rios |
155 |
632 |
.277-.283 |
18-20 |
18-21 |
78-83 |
82-86 |
|
148 |
603 |
0.279 |
19 |
19 |
79 |
83 |
||
7 |
7 |
8 |
7 |
8 |
37 |
|||
B.J. Upton |
155 |
660 |
.245-.250 |
23-26 |
26-30 |
74-78 |
85-89 |
|
3 |
8 |
9 |
7 |
9 |
36 |
|||
Jacoby Ellsbury |
155 |
687 |
.281-.287 |
12.0-16 |
32-37 |
90-95 |
60-65 |
|
135 |
598 |
0.282 |
14 |
32 |
88 |
61 |
||
7 |
5 |
10 |
9 |
5 |
36 |
|||
Curtis Granderson |
155 |
675 |
.238-.244 |
33-37 |
9.0-13 |
95-99 |
92-96 |
|
120 |
557 |
0.248 |
30 |
11 |
88 |
85 |
||
3 |
9 |
7 |
9 |
8 |
36 |
|||
Norichika Aoki |
155 |
694 |
.286-.290 |
13-15 |
24-28 |
93-97 |
56-60 |
|
8 |
5 |
9 |
10 |
4 |
36 |
|||
Alex Gordon |
155 |
695 |
.280-.288 |
15-18 |
8.0-12 |
90-94 |
75-79 |
|
7 |
6 |
6 |
9 |
7 |
35 |
|||
Carlos Beltran |
155 |
635 |
.268-.276 |
18-23 |
7.0-10 |
77-81 |
86-92 |
|
142 |
582 |
0.272 |
20 |
9 |
77 |
88 |
||
6 |
7 |
6 |
7 |
9 |
35 |
|||
Nelson Cruz |
155 |
641 |
.262-.268 |
27-30 |
8.0-11 |
77-81 |
86-92 |
|
135 |
559 |
0.266 |
27 |
10 |
76 |
84 |
||
5 |
9 |
6 |
7 |
8 |
35 |
You can follow Brett on Twitter @TheRealTAL.
[/am4show]
1 Comment
The Xinjiang region’s economy grew by 12 percent in 2012, compared to 2011, but many Uighurs complain that better-paying jobs, land and business opportunities are beyond their grasp. Uighurs account for a little under half of Xinjiang’s 22 million civilian inhabitants; Han Chinese account for 40 percent, according to government data. The Hotan area has about two million inhabitants, nearly 97 percent of them Uighur, according to census data from 2010.